Spin Model for Decision Making
Abstract Decision Making
We aim to understand the fundamental processes behind decision making in the human brain using an Ising-like spin model. Inspired by principles of physics and neuroscience, we represent neurons as interacting spins and simulate neural activity and interactions in the decision-making process. A key aspect of our model is global inhibition, which helps us understand how the brain regulates its overall activity to make accurate decisions.
Our focus is on binary decisions — choosing between two options — and extends to more complex situations involving multiple choices. By modeling these scenarios, we investigate different decision-making regimes and identify the factors that affect decision speed and accuracy. Our goal is to uncover the neural mechanisms that drive these cognitive processes, offering insights into the intricate operations of the brain.

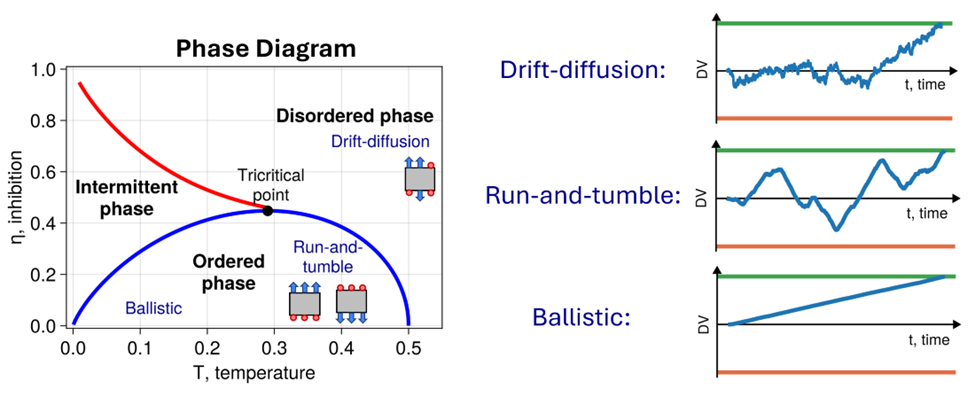
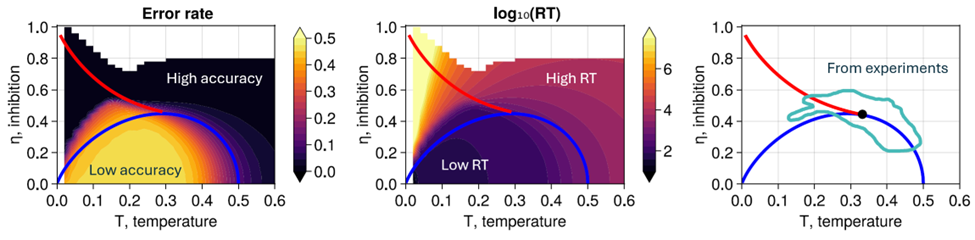
Spatial Decision Making
Shifting the focus to animal behavior, we explore how animals navigate their environment and make decisions on the move. Using an extended version of the same spin model, we simulate how neurons in the brain process spatial information, such as the relative angles between targets, and guide movement. By combining spin dynamics with real-space movement, our model can predict animal trajectories and decision optimality in spatial navigation and during foraging. Our research aims to unify decision-making principles across species and scenarios and bridge the gap between abstract human decisions and the complexities of animal behaviors in different contexts.
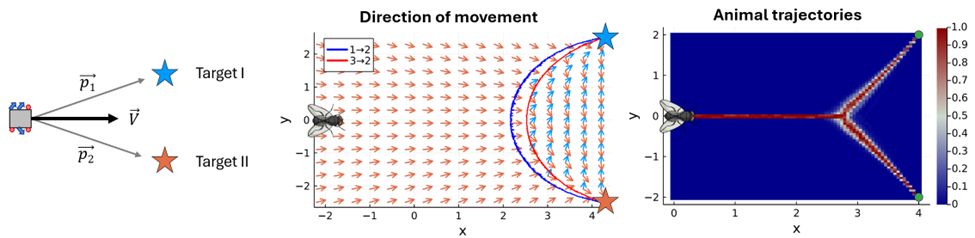
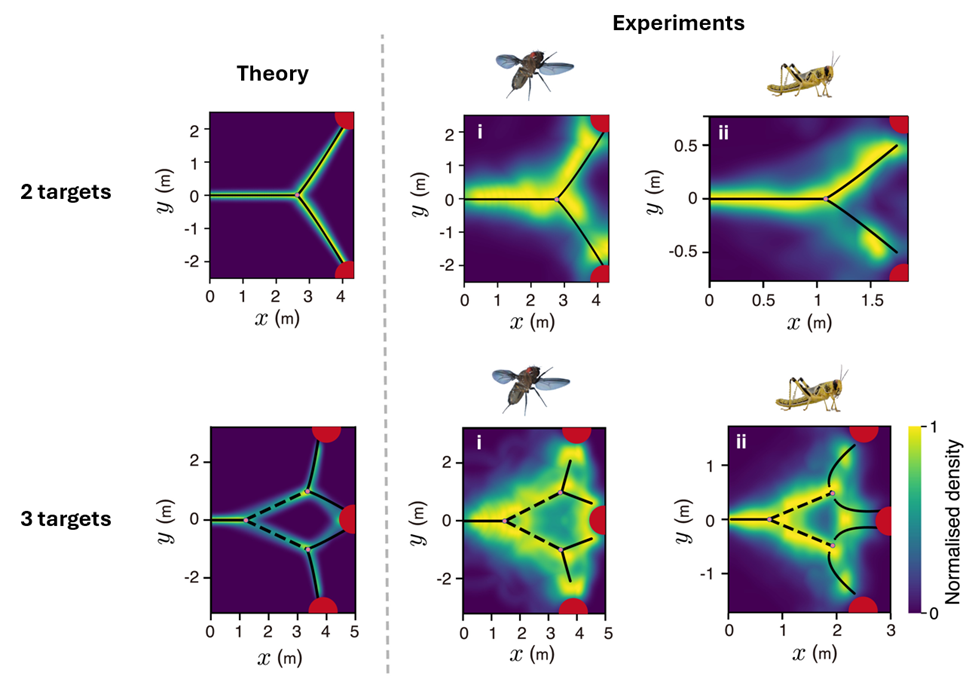
Related papers:
- D. Gorbonos, N. S. Gov, and I. D. Couzin, Geometrical Structure of Bifurcations during Spatial Decision-Making, PRX Life 2, 013008 (2024). DOI: 10.1103/PRXLife.2.013008
- L. Oscar, L. Li, D. Gorbonos, I. D. Couzin, and N. S. Gov, A Simple Cognitive Model Explains Movement Decisions in Zebrafish While Following Leaders, Phys. Biol. 20, 045002 (2023). DOI: 10.1088/1478-3975/acd298
- V. H. Sridhar, L. Li, D. Gorbonos, M. Nagy, B. R. Schell, T. Sorochkin, N. S. Gov, and I. D. Couzin, The Geometry of Decision-Making in Individuals and Collectives, Proc. Natl. Acad. Sci. U.S.A. 118, e2102157118 (2021). DOI: 10.1073/pnas.2102157118
- I. Pinkoviezky, I. D. Couzin, and N. S. Gov, Collective Conflict Resolution in Groups on the Move, Phys. Rev. E 97, 032304 (2018). DOI: 10.1103/PhysRevE.97.032304